Our Research Focus in Responsible Data Science
Our primary mission is AI Safety, incorporating foundational computer science research with broader societal implications of AI.
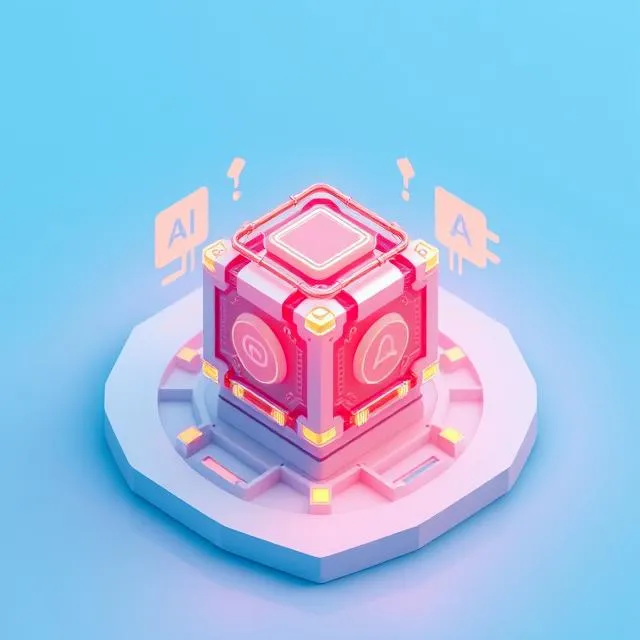
We investigate methods to enhance the resilience of AI models against adversarial influences and dynamic environments. Our work includes:
- Uncertainty Quantification and Robustness: Measuring the confidence of AI models and ensuring the correctness of results.
- Preventing Adversarial Attacks: Understanding and mitigating adversarial threats to AI models.
- Factuality in Large Language Models: Assessing vulnerabilities and improving robustness in generative AI.
- Outlier and Changepoint Detection: Identifying distribution shifts and outliers.
- Visual Object Tracking: Developing reliable tracking systems under challenging real-world conditions.
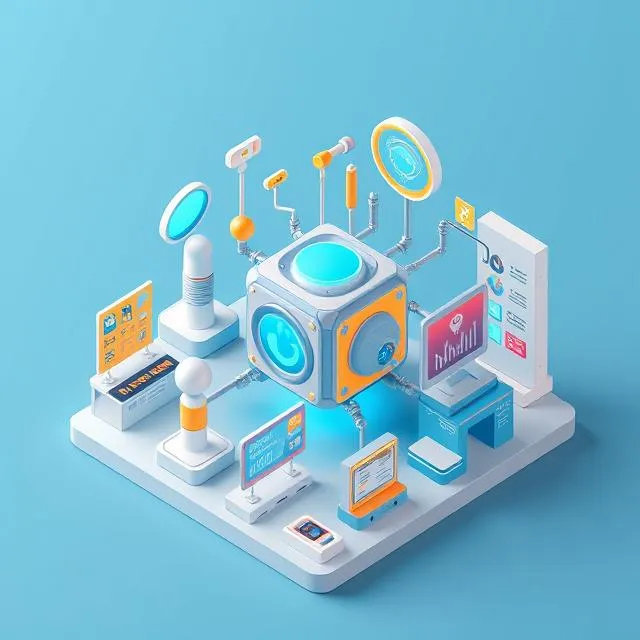
Our research focuses on improving AI’s ability to adapt across diverse contexts and avoid reliance on superficial patterns. Topics include:
- Artificial General Intelligence (AGI): Exploring pathways toward more versatile and adaptable AI systems.
- Bias and Shortcuts: Investigating how AI models develop biases and designing methods to mitigate and counteract them.
- Multi-component Neuro-symbolic Generalization: Combining neural network learning with symbolic reasoning to build AI models with abstraction and logical inference capabilities.
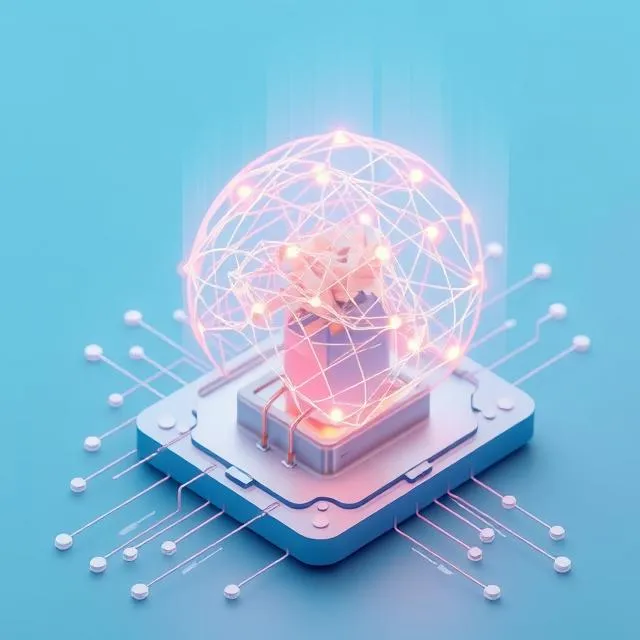
We work on making AI systems more interpretable while ensuring data privacy and ethical compliance. Key areas include:
- Explainable AI: Enhancing model interpretability for users and stakeholders.
- Privacy: Preventing Membership Inference Attacks and improving the practicality of privacy preserving techniques, such as Differential Privacy.
- Machine Unlearning: Developing techniques to selectively remove learned information while preserving model performance.
- Synthetic Data Generation: Creating privacy-preserving realistic datasets for AI training and evaluation.
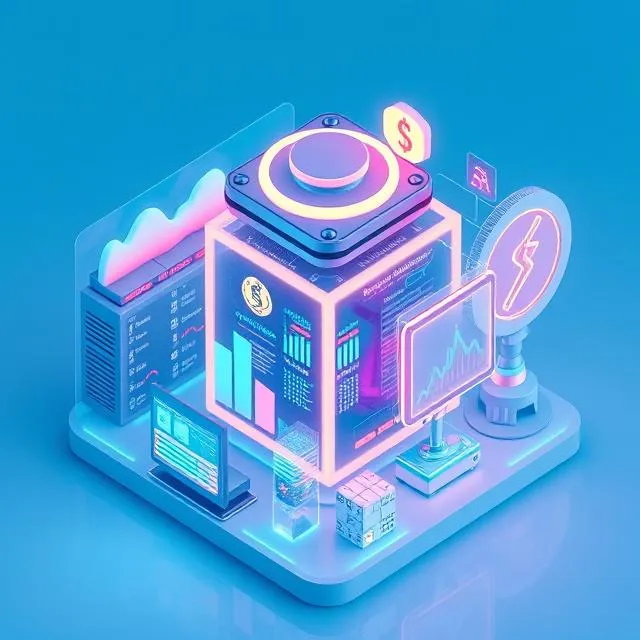
We apply AI research to critical domains, ensuring practical impact and responsible deployment. Our focus includes:
- AI for Finance: Improving risk assessment, fraud detection, and decision-making processes.
- AI for Healthcare: Enhancing diagnostics, patient care, and medical research through AI-driven insights.
- AI in Education: Integrating AI in educational settings and learning analytics.
- AI for Ethics: Refining LLMs to align with ethical guidelines, societal values, and user expectations.
News
- 📄 05/2025: Our paper “Probabilistic Aggregation and Targeted Embedding Optimization for Collective Moral Reasoning in Large Language Models” by Chenchen Yuan, Zheyu Zhang, Shuo Yang, Bardh Prenkaj and Gjergji Kasneci got accepted to ACL Findings 2025. Congratulations to the authors!
- 📄📄📄📄: We are excited to share that RDS has 4 papers accepted at ICML 2025:
- “Grokking in the Wild: Data Augmentation for Real-World Multi-Hop Reasoning with Transformers” by Roman Abramov, Felix Steinbauer, and Gjergji Kasneci.
- “Graph Inverse Style Transfer for Counterfactual Explainability” by Bardh Prenkaj, Efstratios Zaradoukas, and Gjergji Kasneci.
- “SCISSOR: Mitigating Semantic Bias through Cluster-Aware Siamese Networks for Robust Classification” by Shuo Yang, Bardh Prenkaj, and Gjergji Kasneci.
- “Uncertainty Quantification Needs Reassessment for Large Language Model Agents” by Michael Kirchhof, Gjergji Kasneci, and Enkelejda Kasneci. - 📄 04/2025: Our paper “Gender Bias in Explainability: Investigating Performance Disparity in Post-hoc Methods” by Mahdi Dhaini, Ege Erdogan, Nils Feldhus, Gjergji Kasneci was accepted at the ACM Conference on Fairness, Accountability, and Transparency (FAccT) 2025. A preprint is available on arXiv.
- 📄 04/2025: Our paper “EvalxNLP: A Framework for Benchmarking Post-Hoc Explainability Methods on NLP Models” by Mahdi Dhaini, Kafaite Zahra Hussain, Efstratios Zaradoukas, Gjergji Kasneci was accepted at the xAI World Conference (2025) - System Demonstration. A preprint is available on arXiv.
- 🚀 04/2025: Martina Zannotti from the University of Camerino has started her 6 month visiting period at RDS. She is working on Spatio-Temporal Counterfactual Explainability. Welcome Martina!
- 🥳 03/2025: Chenchen Yuan won the TUM EdTech Scholarship 2025. Congratulations!
- 🥳 03/2025: Tobias Leemann graduated with magna cum laude on March 27th, 2025. Congratulations, Dr. Leemann!
- 🏦 03/2025: Bardh Prenkaj won the Friedrich Schiedel Fellowship for Technology in Society. He plans to work on human-readable counterfactual explanations. Congrats!
- 🤝 02/2025: Together with Dino Pedreschi (University of Pisa) and Symeon Papadopoulos (CERTH-ITI), Gjergji Kasneci served on the examination board of Italy’s first national AI for Society doctorates. Big🙏 to Salvatore Ruggieri and many other colleagues for the fantastic organization.
- 🎤 02/2025: At the Munich Young Forum of the Munich Security Conference, Gjergji Kasneci discussed with EU MPs Eva Maydell and Damian von Boeselager, as well as TV journalist Claus Kleber, emerging AI developments and the associated risks in social networks.
- 📣 01/2025: TUM-internal collaboration led by Gjergji Kasneci proposes a blueprint for EU's tech leadership, building on the momentum of the EU AI Action Summit.
- 📄 01/2025: Our paper Attention Mechanisms Don’t Learn Additive Models: Rethinking Feature Importance for Transformers by Tobias Leemann, Alina Fastowski, Felix Pfeiffer, and Gjergji Kasneci was accepted at the Transactions of Machine Learning Research (TMLR). It is available here.
- 📄 11/2024: Our paper “RAZOR: Sharpening Knowledge by Cutting Bias with Unsupervised Text Rewriting” by Shuo Yang, Bardh Prenkaj, and Gjergji Kasneci has been accepted to AAAI 2025. A preprint is available here.
- 🚀 11/2024: Marco Piangerelli and his PhD student, Martina Zannotti, visited us to give talks on their most recent projects: “Unsupervised Explainable Condition Monitoring” and “Exploring Explainability in Spatio-Temporal Graph Neural Networks for Time Series”. We enjoyed it a lot, thanks for stopping by!
- 📣 07/2024: We are happy to announce that our paper "Unifying Evolution, Explanation, and Discernment: A Generative Approach for Dynamic Graph Counterfactuals" by Bardh Prenkaj, Mario Villaizán-Vallelado, Tobias Leemann and Gjergji Kasneci has been accepted as an Oral Presentation at KDD 2024. A preprint is available here.
- 📄 07/2024: Our paper "Understanding Knowledge Drift in LLMs through Misinformation" by Alina Fastowski and Gjergji Kasneci has been accepted at the DELTA workshop at KDD 2024. A preprint is available on arXiv.
- 🚀 07/2024: We had the pleasure to host Giovanni Stilo and Andrea D'Angelo at RDS to present their recent research: “Bias, Fairness, and Explainability: Building Trust in AI Systems” and “Machine Unlearning: Safeguarding Privacy and Security in AI Models”.
- ⚙️ 06/2024: The workshop “DELTA: Discovering Drift Phenomena in Evolving Landscape” proposed by Bardh Prenkaj has been accepted to KDD 2024.
- 🚀 06/2024: Tobias Leemann started a 14-week internship at AWS in New York🗽
- 📄 02/2024: Our paper "Crowdsourcing is breaking my bank! A self-supervised Method for Fine-tuning Pre-trained Language models using Proximal Policy Optimization" by Shuo Yang and Gjergji Kasneci has been accepted at LREC-COLING 2024. A preprint is available on arXiv.
- 📣 01/2024: Our work "I Prefer Not to Say: Protecting User Consent in Models with Optional Personal Data" by Tobias Leemann, Martin Pawelczyk, Christian Thomas Eberle, and Gjergji Kasneci was accepted as an Oral Presentation at AAAI 2024. A preprint is available on arXiv.
- 📄 09/2023: We are happy to announce that our paper "Gaussian Membership Inference Privacy" by Tobias Leemann, Martin Pawelczyk, and Gjergji Kasneci has been accepted for publication at NeurIPS 2023. A preprint is available on arXiv.